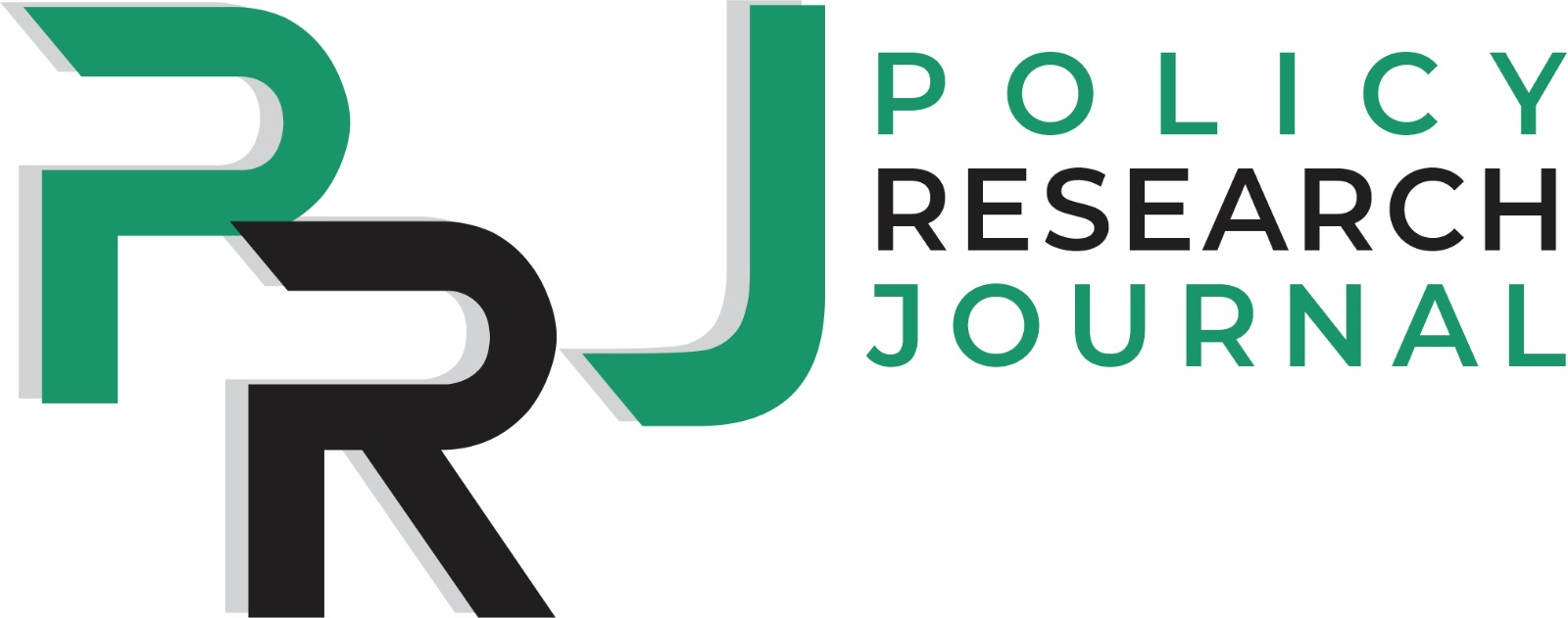
About the Journal
Policy Research Journal (PRJ) , ISSN (E): 3006-7030 ISSN (P) : 3006-7022, published by the Pinnacle Academia Research & Education, serves as a distinguished platform for scholarly discourse. As a multidisciplinary, open-access, and quarterly journal, it stands at the forefront of academic inquiry.
Policy Research Journal upholds rigorous standards of excellence.
Committed to fostering a culture of academic integrity and transparency, Policy Research Journal prioritizes the expeditious completion of the double-blind peer-review process. With an unwavering dedication to scholarly rigor, our team endeavors to provide timely feedback to authors within one month of submission, ensuring a seamless and efficient publication experience.
The journal welcomes papers of the highest quality in the following domains, both established and emerging;
Business & Economics
Education, Language, & Literature
Journalism, Law, & Political Sciences
History & Regional Studies
Psychology & Sociology
Arts & Humanities
Sports Sciences
Engineering
Agriculture Sciences
Earth Sciences
Data Sciences [ Mathematics, Statistics, Computer Science, and Artificial Intelligence]
The respective authors hold copyrights of all content published in the journal.